When IT manager Alex enters the company office on Monday morning, the IT security team is already in turmoil. The new Security Information and Event Management (SIEM) system generated thousands of alerts over the weekend. The sheer volume of data makes it almost impossible to distinguish real threats from false positives. As SIEM collects and correlates security data from various sources, including network devices, servers, databases, applications, endpoints, etc., information remains overwhelming without a clear analysis strategy. In other words: A deep-dive analysis to identify and react to security breaches based on raised alerts is impossible in an acceptable amount of time. The IT security team needs a new strategy to separate the sheep from the goats, as well as to derive rules and automatisms that help accelerate reaction to alert bursts.
How does a cybersecurity strategy look in the era of AI
Leveraging the power of Machine Learning, the IT security team could analyze historical security data to identify patterns and trends allowing to create profiles for normal and unusual behavior. This will enable the team to reduce false positives and focus on real threats. In addition, generative AI helps transform data into understandable information. Wouldn’t it be great if you were able to talk to a security system like to colleague? The IT security team would ask: «Are there any critical threats that require immediate attention?» The system would respond promptly and precisely, listing the major risks and recommending measures.
SIEM’s role in cybersecurity
When it comes to ensuring the security of an organization, solid information gathering and analytical techniques play a key role. A good practice is to forward security-relevant logs to a SIEM system. However, this alone won’t significantly enhance a company’s security if it lacks the ability to correlate and process the information consistently. The maturity level of an organization in terms of security log management can be categorized as follows:
Low: Security-relevant logs are not centralized. Storage policies are based on individual products and projects.
Medium: Security-relevant logs are identified across all products, resources, and projects and are centralized in a SIEM. A global retention policy is defined. This maturity level is crucial for a-posteriori forensic analysis in the event of a major breach or security incident.
High: A triage process is defined within SIEM, and events are correlated. Administrators are notified in case of alerts, and the rate of false positives and false negatives is low.
Expert: A Security Orchestration and Automated Response (SOAR) process is in place. Automated actions are implemented upon detecting an attack or breach, such as account deactivation or endpoint isolation.
Shifting from maturity level «medium» to «high» is a significant undertaking. Reducing false positives without increasing false negatives can be complex. With the emergence of AI in recent years, many products have enhanced SIEM capabilities to improve the accuracy of threat detection based on AI.
The maturity of AI SIEM solutions
The maturity of SIEM solutions based on AI has significantly evolved in recent years.
Machine learning has been used for a while in SIEM products to automate data aggregation and enrich events with additional sources, enabling proactive threat detection and response.
By learning from past security breaches and attack patterns, AI models can predict and detect potential threats before they occur. This significantly reduces the impact of security breaches.
Generative AI is now beginning to further revolutionize SIEM by helping security analysts investigate incidents through natural language interaction without having to ask complex queries. This approach has the potential to massively speed up analysis processes. Another use case is the automation of security operations tasks like incident responses which are based on pre-configured playbooks. Generative AI can suggest and also initiate immediate response measures in order to, e.g., isolate compromised systems, block malicious IPs, trigger user credential resets, or provide summaries of incident and execution reports.
Solutions have transitioned from traditional, rule-based systems to highly sophisticated platforms that leverage AI. These platforms are now integral components of the security tool portfolios of major cloud providers who leverage trillions of signals collected globally to more quickly detect and analyze patterns that may indicate potential threats. Security experts can therefore prepare to interact with co-pilots in the future to respond to incidents and prioritize threats.
How will AI solutions and humans interact in the future?
Generative AI solutions in the area of security are making significant strides towards a promising future, but are not yet fully mature. They perform very well for basic requests and questions. However, complex queries still require substantial expertise to craft the right prompts, analyze results, distinguish true positives from false positives, and draw accurate conclusions. It will thus remain a human-AI interaction that requires security professionals to learn how to accurately formulate their prompts and draw conclusions from AI generated responses.
Authors
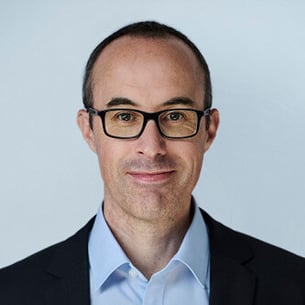
Frederic Kottelat
Security Consultant
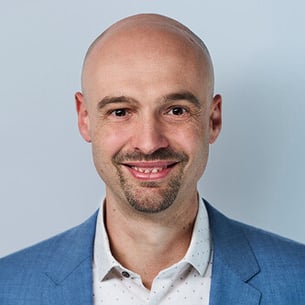
Daniel Hogg
Head of Architecture